The Importance of Bounding Boxes in Data Annotation
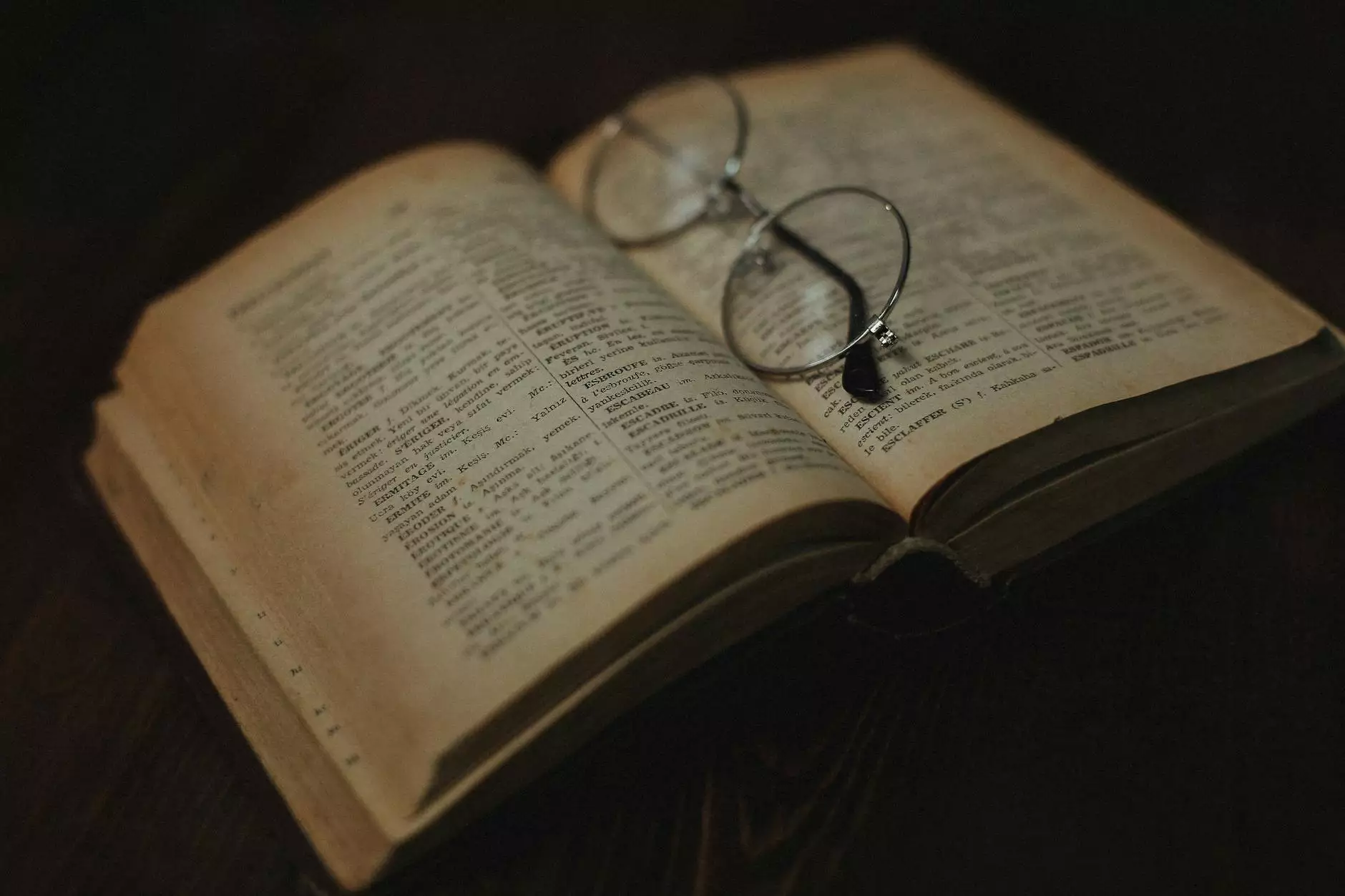
In the realm of data annotation, the term bounding boxes emerges as a pivotal concept, especially for businesses leveraging artificial intelligence (AI) and machine learning (ML) technologies. In this article, we delve deeply into bounding boxes, exploring what they are, their significance in data annotation, and how they facilitate the development of robust and efficient machine learning models.
What are Bounding Boxes?
Bounding boxes are rectangular boxes that are used to identify and localize objects in images or videos. In the context of computer vision, these boxes are drawn around the objects of interest within the training datasets. For instance, if an algorithm needs to detect pedestrians in an image, bounding boxes will outline where each pedestrian is located within the frame.
The Role of Bounding Boxes in Data Annotation
Data annotation is crucial for training machine learning models, especially in areas such as image recognition, autonomous driving, and surveillance systems. Here’s why bounding boxes play an indelible role:
- Object Detection: By providing clear references to what the model is expected to detect, bounding boxes enable precise object localization.
- Improving Accuracy: Annotating data with bounding boxes helps in reducing false positives and negatives during the training process.
- Facilitating Model Training: Well-annotated data with accurate bounding boxes ensures the training phase is more effective, leading to a model that generalizes well on unseen data.
How Bounding Boxes Enhance Machine Learning Models
The integration of bounding boxes into machine learning workflows brings about several benefits:
1. Enhanced Localization
When dealing with complex scenes having multiple objects, accurately defining each object's position is paramount. Bounding boxes act as a clear demarcation, aiding algorithms in understanding the spatial relationships between different entities. This is essential for models designed for human activity recognition, facial recognition, and more.
2. Dataset Optimization
For businesses that collect massive datasets, annotating them with bounding boxes ensures that every element within an image is accounted for. This optimization leads to more relevant training data, ultimately improving the performance of the model.
3. Scalability of Annotation
As businesses scale, the volume of data often increases exponentially. Using bounding boxes allows for a standardized annotation process. Once the criteria are established for drawing these boxes, they can be applied consistently across numerous images, facilitating efficient dataset expansion.
4. Enabling Advanced Models
Modern machine learning techniques, such as convolutional neural networks (CNNs), thrive on well-annotated datasets. The use of bounding boxes not only provides simpler, straightforward object localization but also prepares datasets for more advanced models like YOLO (You Only Look Once) and Faster R-CNN that depend heavily on precise annotation for optimal performance.
Bounding Boxes in Various Applications
Different industries are utilizing bounding boxes in diverse ways:
1. Autonomous Vehicles
Self-driving cars depend on sophisticated perception capabilities to navigate safely. Here, bounding boxes are crucial for recognizing pedestrians, other vehicles, traffic signs, and more.
2. Facial Recognition Systems
In security and surveillance, bounding boxes help identify and track individuals through image feeds. Accurately annotating faces within a dataset enhances the robustness of recognition algorithms.
3. Retail and Inventory Management
In retail environments, smart systems use bounding boxes to monitor inventory levels, recognize customer behavior, and analyze shopping patterns through video feeds.
4. Medical Imaging
In healthcare, bounding boxes can be employed to identify regions of interest within medical images, which aids radiologists in diagnosing conditions and monitoring treatments effectively.
Best Practices for Annotating with Bounding Boxes
- Consistency: Maintain uniform standards for box size and positioning across all annotations.
- Accuracy: Ensure boxes are tightly drawn around the object of interest without cutting it off or including too much extra space.
- Quality Control: Implement a quality control process to review annotations and acknowledge errors or inconsistencies.
- Automation Tools: Utilize advanced annotation tools that can speed up the process while maintaining accuracy.
Choosing the Right Data Annotation Tool
Selecting an appropriate data annotation tool is crucial for businesses. Here are key considerations when evaluating options:
1. User-Friendliness
The tool should have an intuitive interface, making it easy for annotators to create bounding boxes quickly and accurately.
2. Scalability
Your chosen annotation platform should be able to handle varying levels of data volume without compromising on speed or quality.
3. Collaboration Features
As teams grow, collaborative tools that allow multiple users to work on the same dataset can enhance productivity.
4. Integration Capabilities
Evaluate if the annotation platform can integrate with your existing machine learning frameworks to simplify workflows.
Conclusion: The Future of Bounding Boxes in Data Annotation
As AI technologies continue to evolve, bounding boxes will remain a foundational element in the sphere of data annotation. Their ability to enhance machine learning accuracy, improve object detection capabilities, and streamline the annotation process makes them indispensable for businesses across various industries.
Embracing the importance of bounding boxes in data annotation not only positions businesses to harness the full potential of their data but also empowers them to stay competitive in a rapidly changing technological landscape. At Keylabs.ai, we provide cutting-edge data annotation tools and platforms to facilitate your journey into advanced AI applications.