Unlocking the Power of Machine Learning Labeling Tools for Your Business
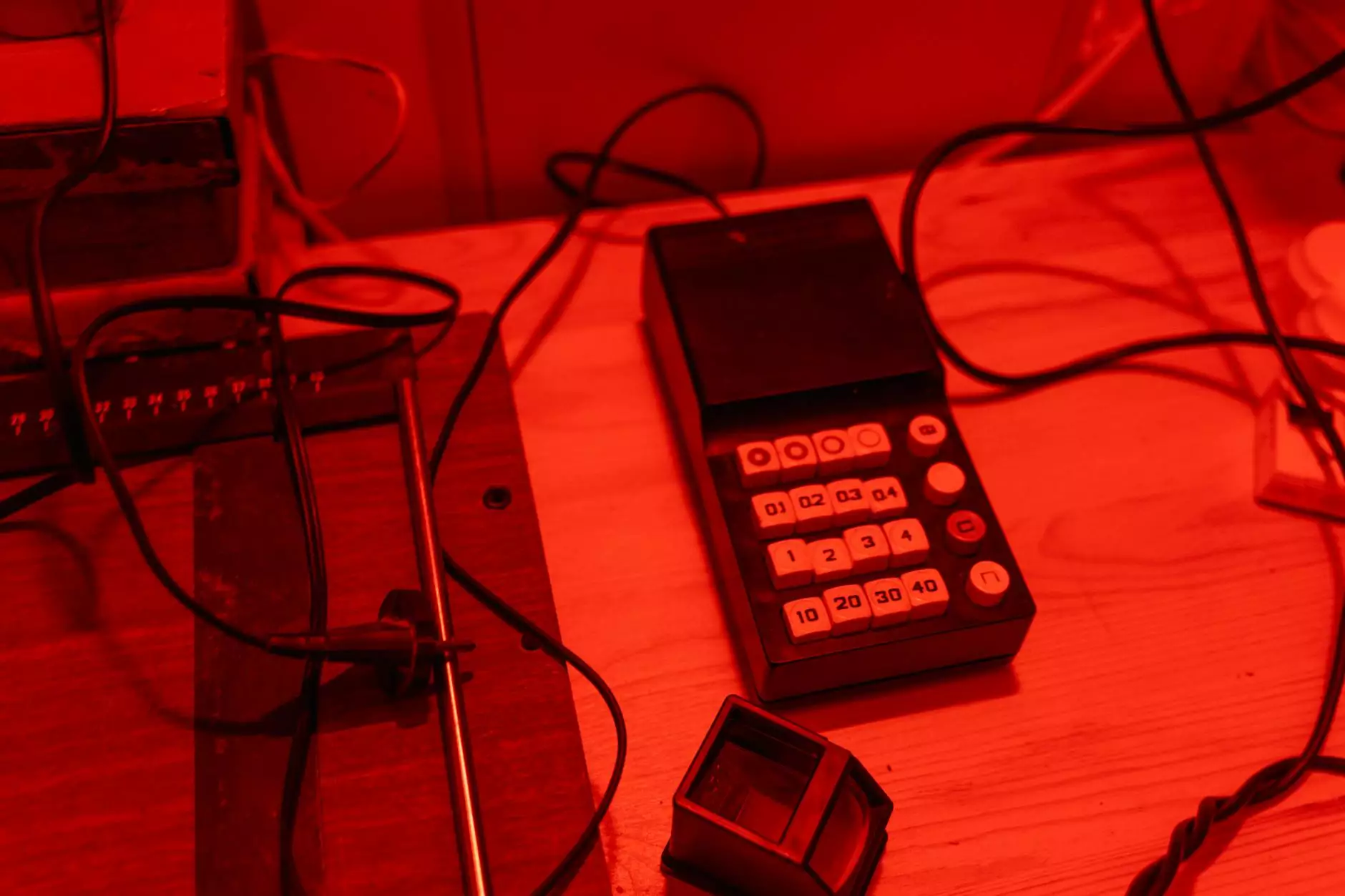
In today’s data-driven world, the significance of machine learning labeling tools cannot be overstated. Businesses are continually seeking innovative ways to harness data to inform decisions, enhance customer experiences, and ultimately drive growth. As we delve into this fascinating domain, we will explore how data annotation tools and platforms, specifically from KeyLabs, can empower organizations to excel in an increasingly competitive landscape.
Understanding Machine Learning and Data Annotation
Machine learning is a branch of artificial intelligence that enables systems to learn from data, identify patterns, and make decisions with minimal human intervention. For machine learning algorithms to be effective, they require vast amounts of high-quality annotated data. This is where data annotation comes into play.
Data annotation refers to the process of labeling data in a way that makes it understandable to machine learning algorithms. This labeled data is crucial for training models to make predictions or classifications accurately. Without the right data annotation tools, businesses may struggle to extract valuable insights or develop robust AI applications.
The Role of Machine Learning Labeling Tools
Machine learning labeling tools facilitate the data annotation process, enabling businesses to annotate data efficiently and accurately. These tools provide a user-friendly interface for annotators to apply labels to datasets, which could include images, text, audio, and video. Here are some key benefits:
- Efficiency: Automating repetitive tasks allows annotators to focus on providing high-quality labels.
- Scalability: Easily manage and scale labeling operations to meet project demands.
- Collaboration: Team members can work together seamlessly, improving workflow and communication.
- Accuracy: Advanced tools ensure precise labeling, reducing the risk of errors and improving model performance.
Key Features of Effective Data Annotation Tools
When selecting a data annotation tool, it is crucial to consider various features that can significantly impact your operations. Here are some essential features to look for:
1. User-Friendly Interface
A well-designed user interface ensures that both novice and experienced annotators can navigate the tool with ease, facilitating quicker and more accurate data annotation.
2. Customizable Labeling Options
The ability to customize labeling tools according to specific project needs is vital. Tools should allow users to define labels, create categories, and organize datasets flexibly.
3. Support for Multiple Data Formats
Data comes in various formats, including text, images, and video. A versatile tool will support all these formats, enabling businesses to work with diverse datasets.
4. Integration Capabilities
Integration with existing systems, such as CRM, data science platforms, or cloud storage solutions, allows seamless data flow and enhances productivity.
5. Quality Control Mechanisms
Implementing mechanisms for quality control, such as review workflows and automated checks, helps maintain high annotation standards, which is essential for successful machine learning outcomes.
6. Collaboration Features
Collaboration tools, including shared dashboards and commenting systems, enhance communication among team members, necessary for large projects.
Benefits of Using KeyLabs Data Annotation Tools
At KeyLabs, we understand the challenges businesses face in data annotation. Our machine learning labeling tools are designed to address these issues effectively. Here are some compelling benefits of using our platform:
- Enhanced Productivity: Our tools streamline the annotation process, reducing the time taken to label datasets efficiently.
- Robust Training Data: Quality assurance protocols ensure that the data produced meets the high standards required for training advanced machine learning models.
- Real-Time Analytics: Users can monitor their projects in real-time, making data-driven decisions to optimize the annotation process.
- Cost-Effectiveness: With improved efficiency and reduced errors, businesses can save costs while maximizing output.
Applications of Machine Learning Labeling Tools
The applications of machine learning labeling tools span various industries, each benefiting from accurate data annotation. Some notable applications include:
1. Healthcare
In healthcare, data annotation tools assist in labeling medical images, enabling the development of diagnostic algorithms that can identify diseases with high accuracy.
2. Autonomous Vehicles
Annotation tools are critical in the automotive industry for mapping the environment, labeling objects, and enhancing computer vision algorithms used in self-driving cars.
3. E-commerce
In e-commerce, machine learning is utilized for personalized recommendations. Annotating product images and descriptions helps train models that understand customer preferences.
4. Finance
In finance, labeling tools facilitate sentiment analysis on financial news articles, market predictions, and risk assessment models, thereby improving decision-making processes.
Choosing the Right Machine Learning Labeling Tool for Your Business
Selecting the right machine learning labeling tool involves assessing your business's specific needs and goals. Here are steps to consider:
- Identify Your Requirements: Understand the type of data you will be annotating and the complexity of the labeling tasks.
- Evaluate Different Tools: Compare various tools based on features, scalability, and user reviews to ensure they meet your needs.
- Consider Support and Training: Look for tools that offer robust customer support and training resources to help your team get started.
- Look for Scalability: Choose a tool that can scale with your business as it grows, accommodating increasing volumes of data.
Future Trends in Machine Learning Labeling Tools
The domain of machine learning labeling tools is continuously evolving. Here are some trends to watch out for in the coming years:
1. Increased Automation
With advances in AI, we can expect more automation in the labeling process, reducing the need for manual intervention and speeding up project timelines.
2. Enhanced Collaboration Tools
Collaboration features will become more sophisticated, streamlining workflows among remote teams working on large-scale projects.
3. Natural Language Processing
Tools that leverage natural language processing (NLP) will improve text annotation methods, enabling better understanding and categorization of textual data.
4. Integration with AI Platforms
Future labeling tools will increasingly integrate with AI development platforms, simplifying workflow and enhancing overall productivity.
Conclusion
Investing in a capable machine learning labeling tool is essential for any business looking to leverage the power of data. Tools like those provided by KeyLabs empower organizations to produce high-quality labeled datasets, driving successful machine learning initiatives. As we move forward into a data-centric future, the importance of efficient data annotation will only grow. By adopting the right tools and strategies, you can ensure that your business stays ahead of the curve, reaping the benefits of artificial intelligence and machine learning technologies.
In summary, as you embark on your machine learning journey, consider how investing in cutting-edge annotation tools will not only streamline your processes but also enhance your overall business outcomes. Embrace the potential of data annotation—your future success may very well depend on it.